Patrick Emedom-Nnamdi
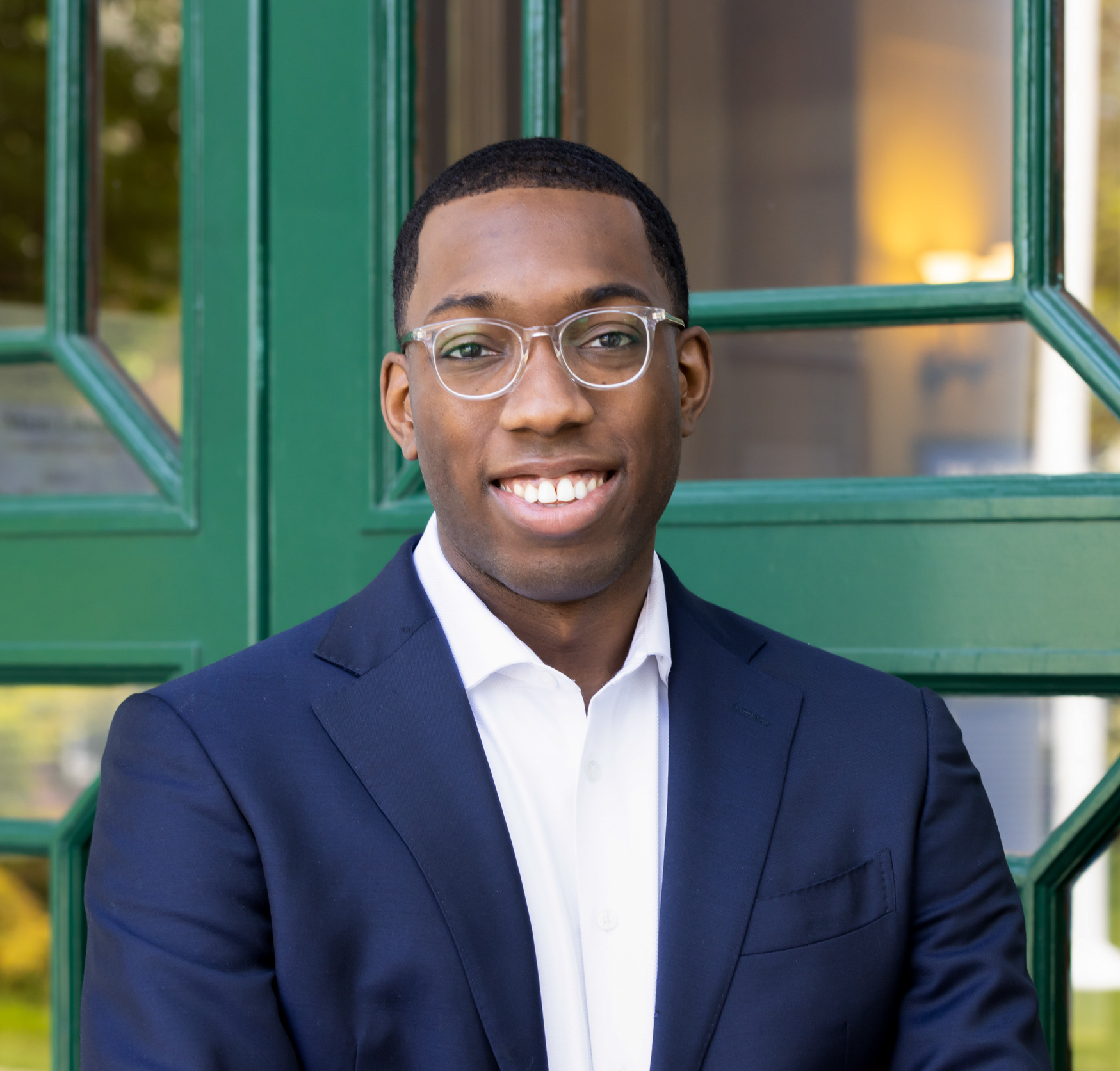
patrickemedom [at] hsph [dot] harvard [dot] edu
I’m a Blavatnik fellow at Harvard University studying the intersection of statistical machine learning and digital phenotyping. My research aims to explore machine learning methods that offer a safe, reliable, and intepretable mechanism for quantifying and improving human quality of life in major clinical applications such as mental health, rehabilitation, and chronic disease management. I’m particularly interested in domains of study operationalized by the ubiquity of continuous data collection via personal digital devices (e.g., smartphones and wearables). These topics include reinforcement learning, state space modeling, and missing data.
I earned a Ph.D. in biostatistics from Harvard under the co-supervision of Dr. Jukka-Pekka Onnela and Dr. Junwei Lu. My Ph.D. was supported by the Harvard Prize Fellowship, the T32 Psychiatric Epidemiology and Biostatistics Grant, and the T32 Cancer Training Grant. I also hold an A.M. in biostatistics from Harvard University and a B.A. in mathematical biology from the University of Pennsylvania.
news
Nov 2023 | Invited participant of Digital Innovations to Improve Maternal and Child Health in Africa |
---|---|
Nov 2023 | Invited participant of Visioning Workshop on Generative mHealth Interventions for Emerging Wearables |
Oct 2023 | Awarded Certificate of Distinction in Teaching (2022-2023 Academic Year) |
May 2023 | Selected as a 2023-2024 Blavatnik Fellow at Harvard Business School! ![]() ![]() |
May 2023 | Officially graduated with a PhD in Biostatistics from Harvard University! For my teaching efforts, I was award a teaching fellow award at graduation. ![]() ![]() ![]() |
May 2023 | Successfully defended my PhD thesis, “Interpretable Statistical Learning for Real-World Behavioral Data”! ![]() ![]() |
Mar 2023 | Participated in the 3rd annual Learning Workshop. |
Mar 2023 | DeepMind Internship paper “Knowledge Transfer from Teachers to Learners in Growing-Batch Reinforcement Learning” accepted at Reincarnating RL Workshop at ICLR 2023. |